Current Projects
AI Tools for Humanitarian Negotiations

This project focuses on designing AI tools to support humanitarian negotiators in high-stakes contexts, such as conflict zones, where decisions impact access to vital resources. We developed a probe interface that generates visualizations like the “Island of Agreements” and the “Stakeholder Iceberg,” enabling negotiators to identify zones of possible agreement and assess risks. These tools augment, rather than replace, human expertise by supporting process-oriented workflows and collaboration while (potentially) mitigating issues like AI overreliance and hallucinations. Insights from this work have been shared in AI in Negotiation Workshops attended by over 100 professionals from organizations like the UN and MSF. Based on our current findings, we are designing new intelligent tools for negotiators to reason about trade-offs during a negotiation.
Supporting Decision Subjects through Contestation
Most human-AI interaction research focuses on supporting the needs of a decision-maker. In this line of work we are interested in supporting the needs of the decision-subject — individuals subject to high-stakes or adverse AI decisions. Our work in this area began with studying algorithmic recourse, a subfield of XAI concerned with providing decision-subjects with explanations indicating how to reverse adverse AI decisions when re-applying for consideration. Our findings suggest a limit to the utility of explanations in supporting decision-subjects within the algorithmic recourse paradigm, leading us to shift attention towards algorithmic contestation, where decision-subjects can address adverse decisions through a wider range of processes than accepting institutions’ initial decisions and re-applying for consideration. To begin understanding how to design socio-technical interventions that support contestation, we conducted formative research on what the situated practice of contestation looks like in contexts across the world, amongst communities on the margins.
Relevant papers
- Counterfactual Explanations May Not Be the Best Algorithmic Recourse Approach. In: Proceedings of the 30th International Conference on Intelligent User Interfaces, ACM Press, New York, NY, USA, 2025.
- Understanding Contestability on the Margins: Implications for the Design of Algorithmic Decision-making in Public Services. In: Proceedings of the 2024 CHI Conference on Human Factors in Computing Systems, Association for Computing Machinery, Honolulu, HI, USA, 2024, ISBN: 9798400703300.
AI-Supported Decision-Making
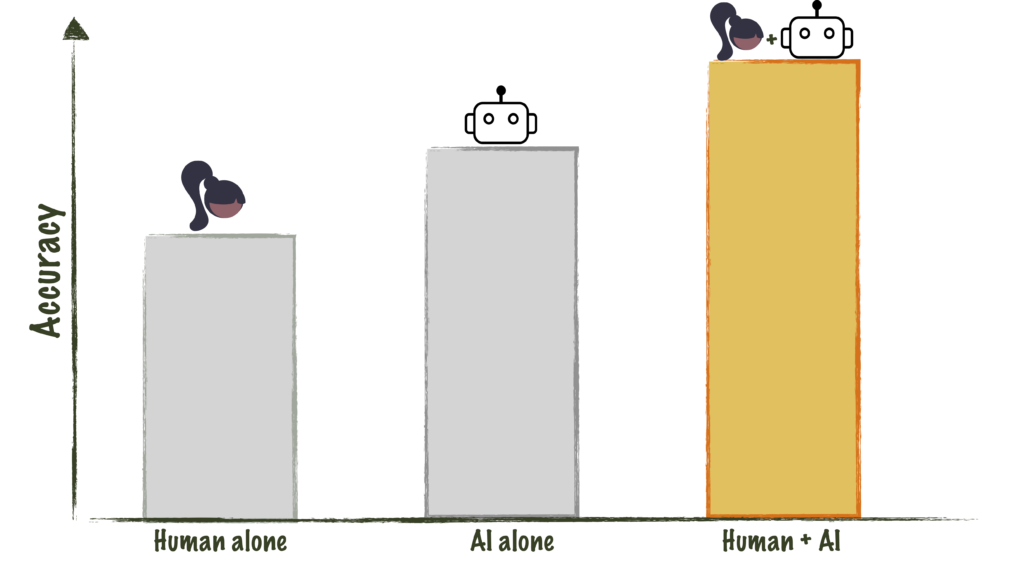
People increasingly interact with AI-powered tools when making decisions or completing tasks. Beyond influencing task-centric outcomes such as decision accuracy and efficiency, we argue that the design of human-AI interactions also impacts human-centric outcomes, including human skills, learning, agency, and collaboration. Our research aims to advance the understanding of human-AI decision-making and to design interaction techniques that optimize both task- and human-centric outcomes.
The first line of work, grounded in cognitive and social science theories, seeks to uncover the principles and mechanisms that govern how people make AI-assisted decisions. For example, contrary to implicit assumptions in the field, our work has demonstrated that people do not consistently engage with each AI recommendation or explanation, and that cognitive engagement moderates human-AI team performance.
The second line of work translates these insights into novel interaction techniques that enhance both task-centric and human-centric outcomes in AI-assisted decision-making. Examples include cognitive forcing interventions that mitigate overreliance on AI, adaptive AI support that enables human-AI complementarity in decision accuracy, and explanations without recommendations, as well as contrastive explanations, that simultaneously improve decision accuracy and people’s learning about the task.
Relevant papers
- Contrastive Explanations That Anticipate Human Misconceptions Can Improve Human Decision-Making Skills. In: Proceedings of the CHI Conference on Human Factors in Computing Systems, ACM, Forthcoming, ISBN: 979-8-4007-1394-1/25/04.
- Personalising AI assistance based on overreliance rate in AI-assisted decision making. In: Proceedings of the 30th International Conference on Intelligent User Interfaces, ACM Press, New York, NY, USA, 2025.
- Accuracy-Time Tradeoffs in AI-Assisted Decision Making under Time Pressure. In: Proceedings of the 29th International Conference on Intelligent User Interfaces, pp. 138–154, Association for Computing Machinery, Greenville, SC, USA, 2024, ISBN: 9798400705083.
- Do People Engage Cognitively with AI? Impact of AI Assistance on Incidental Learning. In: pp. 794–806, Association for Computing Machinery, Helsinki, Finland, 2022, ISBN: 9781450391443.
- To Trust or to Think: Cognitive Forcing Functions Can Reduce Overreliance on AI in AI-Assisted Decision-Making. In: Proc. ACM Hum.-Comput. Interact., vol. 5, no. CSCW1, 2021.
- Designing AI for Trust and Collaboration in Time-Constrained Medical Decisions: A Sociotechnical Lens. In: Proceedings of the 2021 CHI Conference on Human Factors in Computing Systems, Association for Computing Machinery, New York, NY, USA, 2021, ISBN: 9781450380966.
- How machine-learning recommendations influence clinician treatment selections: the example of antidepressant selection. In: Translational Psychiatry, vol. 11, 2021.
- Proxy Tasks and Subjective Measures Can Be Misleading in Evaluating Explainable AI Systems. In: Proceedings of the 25th International Conference on Intelligent User Interfaces, pp. 454–464, Association for Computing Machinery, Cagliari, Italy, 2020, ISBN: 9781450371186.
Rethinking AI for Social Good
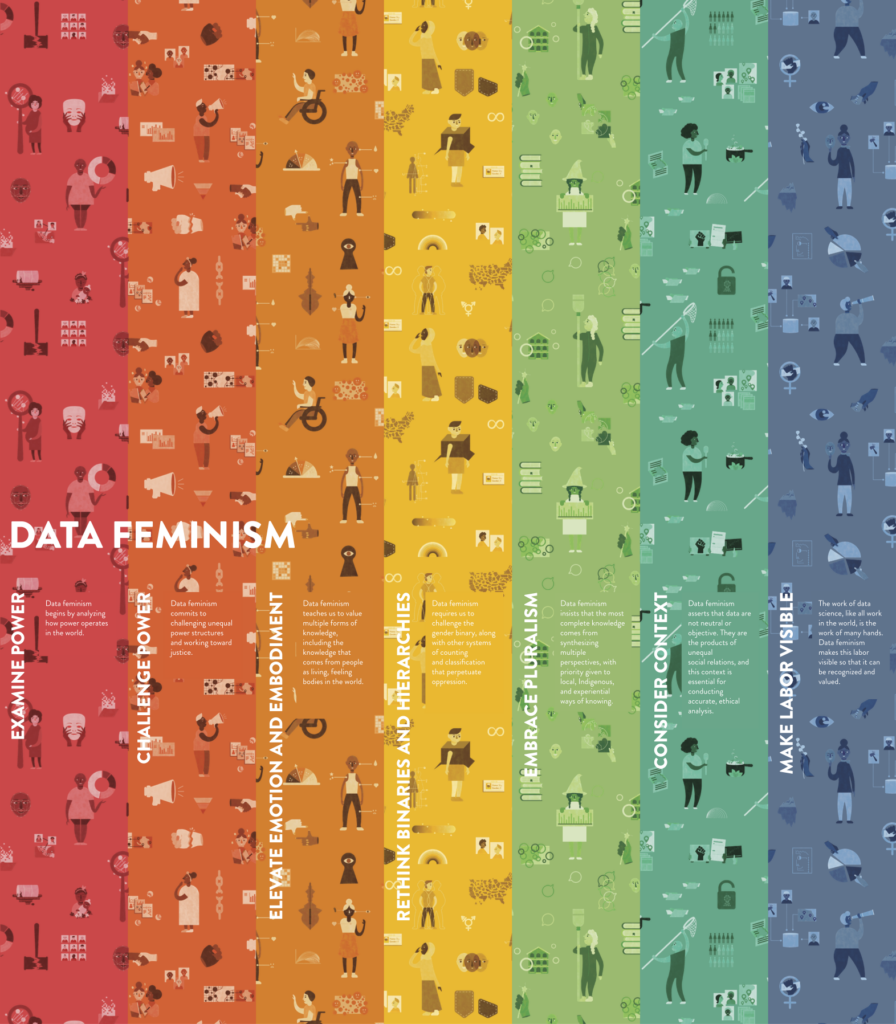
Artificial Intelligence for Social Good (AI4SG) has emerged as a growing body of research and practice exploring the potential of AI technologies to tackle social issues. AI4SG emphasizes interdisciplinary partnerships with community organizations, such as non-profits and government agencies. However, amidst excitement about new advances in AI and their potential impact, power imbalances among AI4SG stakeholders (such as funders, AI teams, and community organizations) and their influence on project priorities and outcomes are not well understood. Our first study in this project investigated community organizations’ needs, expectations, and aspirations. Drawing on the Data Feminism framework, we examined power in AI4SG partnerships and highlighted the pervasive influence of funding agendas and the optimism surrounding AI’s potential, which contributed to community organizations’ goals being frequently sidelined. Building on this finding, we are currently analyzing the funding priorities of AI4SG through a qualitative analysis of funding calls and documents.
Relevant papers
- “Come to us first”: Centering Community Organizations in Artificial Intelligence for Social Good Partnerships. In: Proc. ACM Hum.-Comput. Interact., vol. 8, no. CSCW2, 2024.
Digital Phenotyping of Motor Impairments
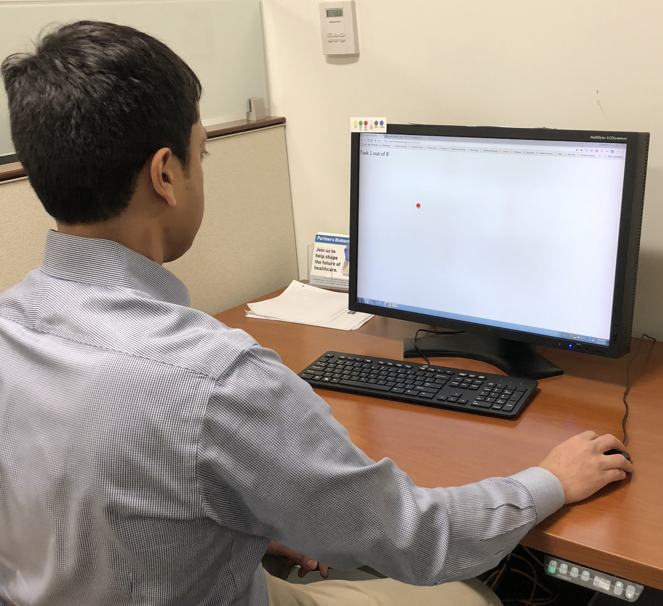
Research on accessible computing as well as healthcare, clinical trials, and neurological disease research all require tools for accurately and objectively measuring motor impairments. Our first tool, called Hevelius, measures motor impairment in the dominant arm based on a person’s performance on a simple computer mouse-based task. We are working on other tools as well as on ways to make accurate measurements possible at home. Such at-home measurements can enable granular longitudinal measurements of disease progression as well as large-scale assessments. This project is done in collaboration with the Laboratory for Deep Neurophenotyping at the Massachusetts General Hospital.
Relevant papers
- Bridging Ontologies of Neurological Conditions: Towards Patient-centered Data Practices in Digital Phenotyping Research and Design. In: Proc. ACM Hum.-Comput. Interact, ACM Press, Forthcoming.
- Hevelius Report: Visualizing Web-Based Mobility Test Data For Clinical Decision and Learning Support. In: Proceedings of the 26th International ACM SIGACCESS Conference on Computers and Accessibility, Association for Computing Machinery, St. John’s, NL, Canada, 2024, ISBN: 9798400706776.
- Accuracy and Reliability of At-home Quantification of Motor Impairments Using a Computer-based Pointing Task with Children with Ataxia-Telangiectasia. In: ACM Transactions on Accessible Computing, vol. 16, no. 1, 2023, ISSN: 1936-7228.
- Real-life ankle submovements and computer mouse use reflect patient-reported function in adult ataxias. In: Brain Communications, vol. 5, no. 2, 2023, ISSN: 2632-1297.
- Free-Living Motor Activity Monitoring in Ataxia-Telangiectasia. In: The Cerebellum, vol. 21, iss. 3, pp. 368–379, 2022.
- Computer Mouse Use Captures Ataxia and Parkinsonism, Enabling Accurate Measurement and Detection. In: Movement Disorders, vol. 35, iss. 2, pp. 354–358, 2020.
Behavior Research at Scale with LabintheWild
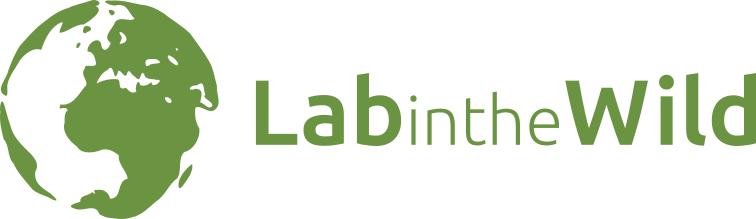
Lab in the Wild is a platform for conducting large scale behavioral experiments with unpaid online volunteers. LabintheWild helps make empirical research in Human-Computer Interaction more reliable (by making it possible to recruit many more participants than would be possible in conventional laboratory studies) and more generalizable (by enabling access to very diverse groups of participants).
LabintheWild experiments at times attract thousands or tens of thousands of participants (with two studies reaching more than 250,000 people). LabintheWild’s volunteer participants have also been shown to provide more reliable data and exert themselves more than participants recruited via paid platforms (like Amazon Mechanical Turk). A key characteristic of LabintheWild is its incentive structure: Instead of money, participants are rewarded with information about their performance and an ability to compare themselves to others. This design choice engages curiosity and enables social comparison—both of which motivate participants.
LabintheWild is co-directed by Profs. Katharina Reinecke at University of Washington and Krzysztof Gajos.
Papers validating LabintheWild
- LabintheWild: Conducting Large-Scale Online Experiments With Uncompensated Samples. In: Proceedings of the 18th ACM Conference on Computer Supported Cooperative Work & Social Computing, pp. 1364–1378, ACM, Vancouver, BC, Canada, 2015, ISBN: 978-1-4503-2922-4.
- Volunteer-Based Online Studies With Older Adults and People with Disabilities. In: Proceedings of the 20th International ACM SIGACCESS Conference on Computers and Accessibility, pp. 229–241, ACM, Galway, Ireland, 2018, ISBN: 978-1-4503-5650-3.
- Conducting online virtual environment experiments with uncompensated, unsupervised samples. In: PLOS ONE, vol. 15, no. 1, pp. 1–17, 2020.
Some papers using data collected on LabintheWild
- Sex and age differences in “theory of mind” across 57 countries using the English version of the “Reading the Mind in the Eyes” Test. In: Proceedings of the National Academy of Sciences, vol. 120, no. 1, pp. e2022385119, 2023.
- Do People Engage Cognitively with AI? Impact of AI Assistance on Incidental Learning. In: pp. 794–806, Association for Computing Machinery, Helsinki, Finland, 2022, ISBN: 9781450391443.
- Computer Mouse Use Captures Ataxia and Parkinsonism, Enabling Accurate Measurement and Detection. In: Movement Disorders, vol. 35, iss. 2, pp. 354–358, 2020.
- The Influence of Personality Traits and Cognitive Load on the Use of Adaptive User Interfaces. In: Proceedings of the 22Nd International Conference on Intelligent User Interfaces, pp. 301–306, ACM, Limassol, Cyprus, 2017, ISBN: 978-1-4503-4348-0.
- The Role of Explanations in Casual Observational Learning About Nutrition. In: Proceedings of the 2017 CHI Conference on Human Factors in Computing Systems, pp. 4097–4145, ACM, Denver, Colorado, USA, 2017, ISBN: 978-1-4503-4655-9.
- Learning From the Crowd: Observational Learning in Crowdsourcing Communities. In: Proceedings of the 2016 CHI Conference on Human Factors in Computing Systems, pp. 2635–2644, ACM, Santa Clara, California, USA, 2016, ISBN: 978-1-4503-3362-7.
- Quantifying Visual Preferences Around the World. In: Proceedings of the SIGCHI Conference on Human Factors in Computing Systems, pp. 11–20, ACM, Toronto, Ontario, Canada, 2014, ISBN: 978-1-4503-2473-1.
- Predicting users’ first impressions of website aesthetics with a quantification of perceived visual complexity and colorfulness. In: Proceedings of the SIGCHI Conference on Human Factors in Computing Systems, pp. 2049–2058, ACM, Paris, France, 2013, ISBN: 978-1-4503-1899-0.
Past projects
Improving Care Coordination in Complex Healthcare
Children with complex health conditions require care from a large, diverse team of caregivers that includes multiple types of medical professionals, parents and community support organizations. Coordination of their outpatient care, essential for good outcomes, presents major challenges. Our formative studies revealed that the nature of teamwork in complex care poses challenges to team coordination that extend beyond those identified in prior work and that can be handled by existing coordination systems. We are building on a computational theory of teamwork to create entirely new tools to support complex, loosely-coupled teamwork.
Relevant papers
- “I Think We Know More Than Our Doctors”: How Primary Caregivers Manage Care Teams with Limited Disease-related Expertise. In: Proc. ACM Hum.-Comput. Interact., vol. 3, no. CSCW, pp. 159:1–159:22, 2019, ISSN: 2573-0142.
- Personalized change awareness: Reducing information overload in loosely-coupled teamwork. In: Artificial Intelligence, vol. 275, pp. 204 – 233, 2019, ISSN: 0004-3702.
- Mutual Influence Potential Networks: Enabling Information Sharing in Loosely-Coupled Extended-Duration Teamwork. In: Proceedings of IJCAI’16, 2016.
- From Care Plans to Care Coordination: Opportunities for Computer Support of Teamwork in Complex Healthcare. In: Proceedings of the 33rd Annual ACM Conference on Human Factors in Computing Systems, pp. 1419–1428, ACM, Seoul, Republic of Korea, 2015, ISBN: 978-1-4503-3145-6.
- AI Support of Teamwork for Coordinated Care of Children with Complex Conditions. In: AAAI Fall Symposium: Expanding the Boundaries of Health Informatics Using AI: Making Personalized and Participatory Medicine A Reality, 2014.
Impact of Predictive Text on the Content of What People Write
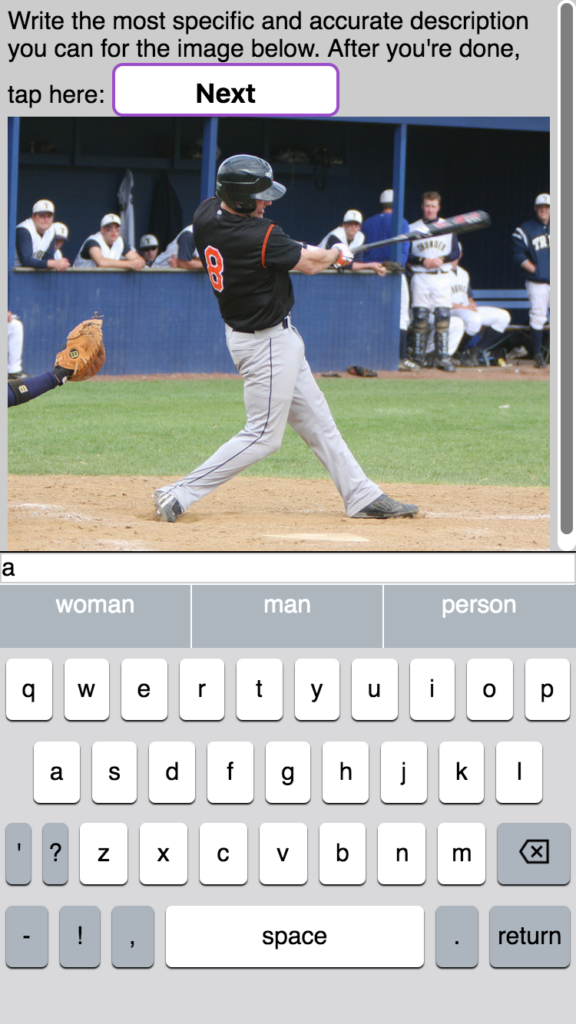
Predictive text technology (e.g., the word suggestions displayed on most keyboards on mobile devices) was designed to improve the ease and efficiency of text entry. Our work demonstrates that predictive text can influence the content of what people write. Specifically, predictive text tools appear to cause people to make word choices that are more “predictable” given the model used by predictive text and using fewer adjectives or other embellishments compared to writing without predictive text. Moreover, in our 2018 paper, manipulating sentiment bias of the model powering predictive text entry impacted the sentiment of the restaurant reviews that people wrote even though they had committed to a star rating for that review prior to beginning to write.
Relevant papers
- Predictive Text Encourages Predictable Writing. In: Proceedings of the 25th International Conference on Intelligent User Interfaces, pp. 128–138, Association for Computing Machinery, Cagliari, Italy, 2020, ISBN: 9781450371186.
- Sentiment Bias in Predictive Text Recommendations Results in Biased Writing. In: Proceedings of Graphics Interface 2018, pp. 33 – 40, Canadian Human-Computer Communications Society / Societe canadienne du dialogue humain-machine, Toronto, Ontario, 2018, ISBN: 978-0-9947868-2-1.
- On Suggesting Phrases vs. Predicting Words for Mobile Text Composition. In: Proceedings of the 29th Annual Symposium on User Interface Software and Technology, pp. 603–608, ACM, Tokyo, Japan, 2016, ISBN: 978-1-4503-4189-9.
DERBI: Communicating Individual Biomonitoring and Personal Exposure Results to Study Participants
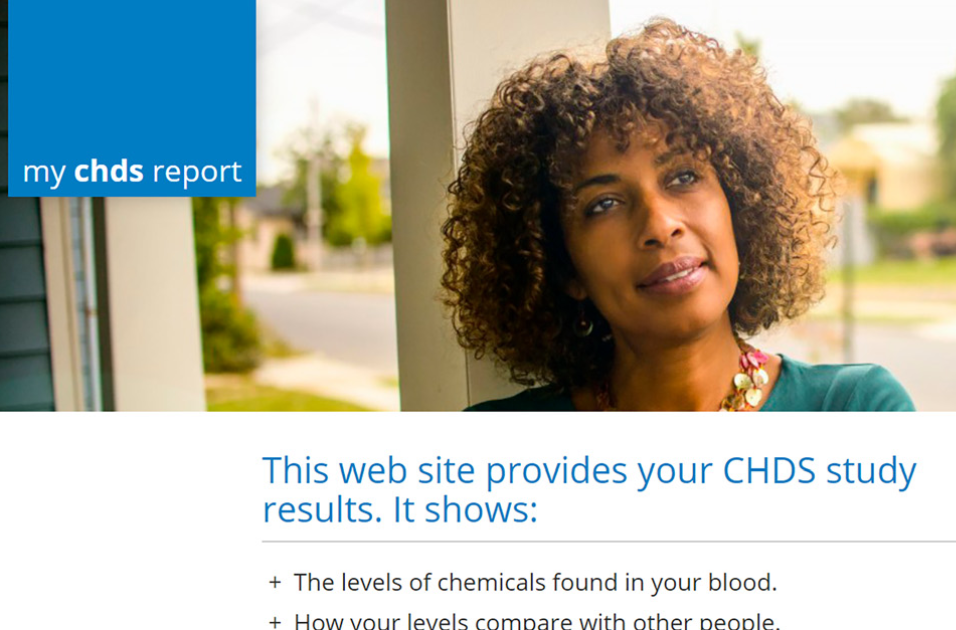
Epidemiologic studies and public health biomonitoring rely on chemical exposure measurements in blood, urine, and other tissues, and in personal environments, such as homes. For many chemicals, the health implications of individual results are uncertain, and the sources and strategies to reduce exposure may not be known. Yet, a growing number of researchers consider it their ethical obligation to report the results back to their participants. In a project led by the Silent Spring Institute, we are building scalable online tools to help researchers communicate personalized results to study participants in a manner that appropriately conveys what is and what is not known about the sources and effects of different environmental chemicals.
Relevant papers
- Outcomes from Returning Individual versus Only Study-Wide Biomonitoring Results in an Environmental Exposure Study Using the Digital Exposure Report-Back Interface (DERBI). In: Environmental health perspectives, vol. 129, no. 11, pp. 117005, 2021.
- DERBI: A Digital Method to Help Researchers Offer “Right-to-Know” Personal Exposure Results. In: Environmental Health Perspectives, vol. 125, no. 2, 2017.